Speakers and Summaries
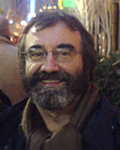 |
Daniel Bennequin
Professor, Institut de Mathématiques de Jussieu, Université Paris Diderot, France
|
Geometrical invariance in movement generation
The presentation will describe how the notion of geometrical invariance, specially affine invariance, can help to understand the preparation, generation and control of voluntary movements. In addition, we suggest that several geometries are used by the brain for producing hand and locomotion trajectories with their durations. This accords to principles stated by Helmoltz, Poincaré, Piaget, Gibson, Berthoz. Experimental works supporting the theory will be shown, on human locomotion and drawing (with T.Flash, A.Berthoz, F.Polyakov, R.Fuchs, Y.Meirovitch and Q-C. Pham). Recent work of Q-C.Pham and Y.Nakamura shows the interest of this approach for robotics. Finally, we will present current work with Alain Berthoz exploring what could be the geometries underlying different categories of actions.
|
Daniel Bennequin is graduated from Ecole Normale Supérieure. He defended his PHD in 1982 with Alain Chenciner at Paris VII (Entrelacements et équations de Pfaff). IBM prize in 1983. He was Professor at Strasbourg University and was member of Bourbaki. He is currently a Professor at Diderot University, Paris VII, and member of the Institut Mathématique de Jussieu. He has made contributions to contact geometry and knot theory during the 1980’s and was initiator of contact topology with Yakov Eliashberg. During the years 1990, he worked with students and colleagues in Strasbourg, on integrable systems and geometrical structures of Mathematical Physics, a subject he is working again today. Since 2000 he has been working in Neurosciences (mainly with Alain Berthoz, C-d-F, Paris, and Tamar Flash, Weizmann Insitute, Rerhovot, Israel); he made contributions to the study of geometrical invariance in human movements duration, dynamical structure of vestibular end sensors, organization of vestibular information flow, eye movements preparation and gaze functions during locomotion. In 2015, he has published with Pierre Baudot (Max Planck, Leipzig) the elements of a new theory of information topology, and with Michèle Bompard-Porte a book on psychic pains.
|
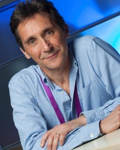 |
Antonio Bicchi
Professor, Italian Institute of Technology, University of Pisa, Genoa, Italy.
|
Artificial hands: from humans to robots and back
In this talk Professor Antonio Bicchi will discuss the reasons behind the long history of beautiful and sophisticated artificial hands which had little impact on affordable and usable devices for robotics or prosthetics. He will argue that the real challenge in hand design is not just to deploy more or better actuators, sensors and algorithms, but to deeply investigate what is really at the core of the hand functionalities, and develop a principled design approach to simplification, thus realizing that often ``simpler'' is by no means ``easier''.
With such motivations, he will discuss recent results and developments of the Pisa-IIT SoftHand, a robot hand prototype designed with the purpose of being as easily usable, robust and simple as possible, while exhibiting a level of grasping versatility and an aspect comparable to that of the human hand. He will briefly review the main theoretical tools used to enable such simplification, i.e. the notion of ``soft synergies''. Of particular relevance in its design is the very soft and safe, yet powerful and extremely robust structure, obtained through the use of innovative articulations and ligaments replacing conventional joint design. Recent work on transferring the Pisa-IIT SoftHand technology to prosthetics will also be introduced.
|
Antonio Bicchi is Senior Scientist with the Italian Institute of Technology in Genoa and Professor of Robotics at the University of Pisa. He graduated from the University of Bologna in 1988 and was a postdoc scholar at MIT AI Lab in 1988–1991. His main research interests are in Robotics, Haptics, and Control Systems. He is Editor-in-Chief of the IEEE Robotics and Automation Letters, and of the Springer series ``Briefs on Control, Automation and Robotics''. He has organized and chaired several international conferences, including the first WorldHaptics Conference (WHC'05), the Int. Symp. on robotics Research (ISRR'15), and the Program Committee of the Int. Conf. Robotics and Automation (ICRA'16). He is the recipient of several awards and honors, including an Advanced Grant from the European Research Council for his research on human and robot hands.
|
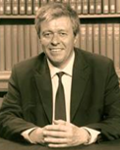 |
Karl Friston
Professor, University College London, Institute of Neurology, London, UK.
|
The variational foundations of movement
This overview of the variational free energy principle offers an account of embodied exchange with the world that associates action with inferring the causes of our sensations. Its agenda is to link formal (mathematical) descriptions of dynamical systems to a description of action in terms of beliefs and goals. The argument has two parts: the first calls on the lawful dynamics of any (weakly mixing) ergodic system– from a single cell organism to a human brain. These lawful dynamics suggest that (internal) states can be interpreted as modelling or predicting the (external) causes of sensory fluctuations. In other words, if a system exists, its internal states must encode probabilistic beliefs about external states. Heuristically, this means that if I exist (am) then I must have beliefs (think). The second part of the argument is that the only tenable beliefs I can entertain about myself are that I exist. This may seem rather obvious; however, if we associate existing with ergodicity, then (ergodic) systems that exist by predicting external states can only possess prior beliefs that their environment is predictable. It transpires that this is equivalent to believing that the world – and the way it is sampled – will resolve uncertainty about the causes of sensations. We will conclude by looking at the epistemic behaviour that emerges under these beliefs, using simulations of movement, action observation and oculomotor searches.
|
Karl Friston is a theoretical neuroscientist and authority on brain imaging. He invented statistical parametric mapping (SPM), voxel-based morphometry (VBM) and dynamic causal modelling (DCM). These contributions were motivated by schizophrenia research and theoretical studies of value-learning – formulated as the dysconnection hypothesis of schizophrenia. Mathematical contributions include variational Laplacian procedures and generalized filtering for hierarchical Bayesian model inversion. Friston currently works on models of functional integration in the human brain and the principles that underlie neuronal interactions. His main contribution to theoretical neurobiology is a free-energy principle for action and perception (active inference). Friston received the first Young Investigators Award in Human Brain Mapping (1996) and was elected a Fellow of the Academy of Medical Sciences (1999). In 2000 he was President of the international Organization of Human Brain Mapping. In 2003 he was awarded the Minerva Golden Brain Award and was elected a Fellow of the Royal Society in 2006. In 2008 he received a Medal, Collège de France and an Honorary Doctorate from the University of York in 2011. He became of Fellow of the Society of Biology in 2012 and received the Weldon Memorial prize and Medal in 2013 for contributions to mathematical biology.
|
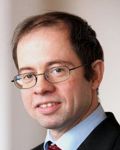 |
Martin A. Giese
Professor, Hertie Institute for Clinical Brain Research and Centre for Integrative Neurocience, University Clinic Tuebingen, Germany
|
Modelling of coordinated human body motion by learning of structured dynamic representations
The modeling of complex coordinated human movements and the interactive movements of multiple humans is essential for the understanding of motor control, and for different technical applications including robotics. The talk presents two approaches for the modeling of coordinated human movements that link the analysis of human motion with technical applications. The first one exploits anechoic demixing in order to approximate complex body movements by combinations of small numbers of movement primitives. These primitives are mapped onto dynamic primitives, which are embedded in dynamic architectures that generate complex coordinated movements online, in a highly adaptive manner. The second approach represents interactive full-body movements by a hierarchical probabilistic architecture, which combines GP-LVMs and GPDMs. It is demonstrated how such architectures can be exploited for the online planning of movements in humanoid robots and for the study of embodied perception in humans.
|
Martin A. Giese (PhD in Electrical Engineering), after a postdoc at CBCL (MIT), headed the HONDA Cambridge Research Laboratory in Boston. He founded the Laboratory for Action Representation and Learning at the Hertie Institute and the University Clinic T¸bingen (Germany) in 2001. After a position as Senior Lecturer in the Dept. of Psychology at the University of Bangor (UK), in 2008 he accepted a position as Professor for Computational Sensomotorics in the Werner Reichardt Center for Integrative Neuroscience, the Hertie Institute for Clinical Brain Research and the University Clinic Tübingen, Germany. His research focuses on the role of learning in action recognition and control in biological systems, and technical applications.
|
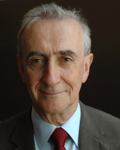 |
Neville Hogan
Professor, Massachusetts Institute of Technology, Newman Laboratory, Cambridge, USA.
|
Optimal physical interaction via dynamic primitives
Humans out-perform contemporary robots despite vastly slower ‘hardware’ (e.g. muscle) and ‘wetware’ (e.g. neurons). This is likely due to control based on dynamic primitives, which enable highly dynamic behavior with minimal high-level supervision and intervention. Including mechanical impedance facilitates controlling physical interaction. It is also a dynamic primitive in the sense that it may emerge from nonlinear neuro-mechanical dynamics. I will review how specifying appropriate mechanical impedance may be cast as an optimization problem, provided the objective function includes both force and motion at an interaction port. I will review how a nonlinear generalization of the classical equivalent circuit serves to combine both motion and interaction primitives and highlights the contrast between information-processing and energy-processing domains.
|
Neville Hogan is Professor of Mechanical Engineering, Professor of Brain and Cognitive Sciences and Director of the Newman Laboratory for Biomechanics and Human Rehabilitation at the Massachusetts Institute of Technology. He is a co-founder of Interactive Motion Technologies, Inc., and a board member of Advanced Mechanical Technologies, Inc. Born in Dublin, Ireland, he earned a Dip. Eng. (with distinction) from Dublin College of Technology and M.S., M.E. and Ph.D. degrees from MIT. Following industrial experience in engineering design, he joined MIT’s School of Engineering faculty in 1979 and has served as Head and Associate Head of the Mechanical Engineering department’s System Dynamics and Control division. Awards include an Honorary Doctorate from the Delft University of Technology; the Silver Medal of the Royal Academy of Medicine in Ireland; and an Honorary Doctorate from the Dublin Institute of Technology. Professor Hogan’s research is broad and multi-disciplinary, extending from biology to engineering—it has made significant contributions to motor neuroscience, rehabilitation engineering and robotics—but its focus converges on an emerging class of machines designed to cooperate physically with humans. Recent work pioneered the creation of robots sufficiently gentle to provide physiotherapy to frail and elderly patients recovering from neurological injury such as stroke, a novel therapy that has already proven its clinical significance.
|
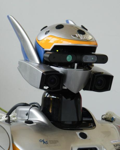 |
HRP-2 14
Humanoid Robot
|
HRP-2 is the robotic platform for the Japanese Humanoid Robotics Project (HRP). The robot was designed and integrated by Kawada Industries, Inc. together with the Humanoid Research Group of the National Institute of Advanced Industrial Science and Technology (AIST). The external appearance of HRP-2 was designed by Mr. Yutaka Izubuchi, a mechanical animation designer. HRP-2’s height is 154 cm and its mass is 58 kg, including batteries. It has 30 degrees of freedom (DOF). About twenty copies of the robot exist today. Only one (HRP2-14) left Japan to serve as a research platform for the robotics teams at LAAS-CNRS in Toulouse. CNRS acquired HRP2-14 in 2005 in the framework of the joint French-Japanese laboratory JRL. |
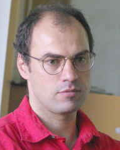 |
Frédéric Jean
Professor, Professor at École Nationale Supérieure de Techniques Avancées ParisTech, France.
|
Why don't we move slower? The value of time in the neural control of action
To want something now rather than later is a common attitude that reflects the brain’s tendency to value the passage of time. Because the time taken to accomplish an action delays task achievement and reward acquisition, this idea was ported to neural movement control within the “cost of time” theory. This theory provides a normative framework to account for the principles governing movement time formation within the brain and the origin of a self-selected pace in human and animal motion. Then, how does the brain exactly value time in the control of action? To resolve this issue, we employed an inverse optimal control approach and developed a complete methodology allowing to sample infinitesimal values of the time cost squarely from experimental motion data. The cost of time underlying saccades was found to have a concave growth, thereby confirming previous results and illustrating the effectiveness of the methodology. For self-paced reaching, however, movement time was valued according to a striking sigmoid-like shape; its rate of change presented a steep rise before a maximum was reached and a slower decay was observed. Properties of uniqueness and robustness of the inferred cost of time were established for the class of problems under investigation, thus reinforcing the significance of the present findings. The derived methodology may have impacts in fields where optimal choice or prediction of motion pace is relevant. In neuroscience, it may offer a unique opportunity to uncover how the brain values the passage of time in healthy and pathological motor control.
|
Frédéric Jean is a mathematician. He is Professor at ENSTA ParisTech, France (member of University Paris-Saclay). He received the Ph.D. in Mathematics and the Habilitation à Diriger des Recherches from the University Pierre et Marie Curie at Paris in 1998 and 2007 respectively.His research activities are concerned with nonlinear control theory, more specifically with geometric control: the objective is to address the typical issues of automatics and control systems by using methods and tools of differential geometry. His researchs focus firstly on theoretical aspects such as sub-Riemannian geometry, non-holonomic systems, geometric approach to optimal control; and secondly on modelling neurophysiology based on control systems, in particular through inverse optimal control problems.
|
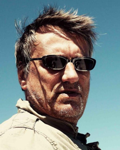 |
Jean-Bernard Lasserre
Directeur de Recherche, CNRS-LAAS, Toulouse, France.
|
The moment-SOS approach for global optimization and optimal control
We will describe the moment-SOS approach for solving the Generalized Moment Problem (GMP), which consists of solving a hierarchy of convex relaxations of the original problem. Each convex relaxation is a semidefinite program which can be solved efficiently (but its size increases in the hierarchy). We provide two examples of applications of this general methodology: Global optimization & Optimal control which indeed can be viewed as two instances of the GMP.
|
Jean B. Lasserre got his "Doctorat d'Etat" from the University of Toulouse in 1984 and is currently ``CNRS Directeur de Recherche" at LAAS in Toulouse as well as an associate member of IMT (the Institute of Mathematics of Toulouse). He has been working in the area of optimization (& Markov control processes) in a broad sense and is crrently interested in applying results & tools from real-algebraic geometry in this area. SIAM Fellow he is the recipient of the 2009 Lagrange prize in Optimization (awarded every 3 years jointly by SIAM and the Mathematical Optimization Society). He is also the author or co-author of 8 books (Birkhauser, Springer-Verlag, Imperial College Press and Cambridge University Press) and numerous publications in international journals.
|
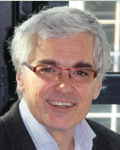 |
Jean-Paul Laumond
Directeur de Recherche, CNRS-LAAS, Toulouse, France.
|
Workshop Opening
While actions operate in a physical space, motions begin in a motor control space. For robots and living beings, the link between actions expressed in the physical space and motions originated in the motor space, turns to geometry in general and, in particular, to linear algebra. The idea to express robot actions as motions to be optimized has been developed in robotics since the 1970s. Geometric control theory and numerical analysis highlight two complementary perspectives on optimal human and humanoid motion. Among all possible motions performing a given action, optimization algorithms tend to choose the best motion according to a given performance criterion. Optimal motions then appear as plausible action signatures.
|
Jean-Paul Laumond, IEEE Fellow, is a roboticist. He is Directeur de Recherche at LAAS-CNRS (team Gepetto) in Toulouse, France. His research is devoted to robot motion. In the 90's, he has been the coordinator of two European Esprit projects PROMotion (Planning RObot Motion) and MOLOG (Motion for Logistics), both dedicated to robot motion planning and control. In the early 2000's he created and managed Kineo CAM, a spin-off company from LAAS-CNRS devoted to develop and market motion planning technology. Kineo CAM was awarded the French Research Ministery prize for innovation and enterprise in 2000 and the third IEEE-IFR prize for Innovation and Entrepreneurship in Robotics and Automation in 2005. Siemens acquired Kineo CAM in 2012. In 2006, he launched the research team Gepetto dedicated to Human Motion studies along three perspectives: artificial motion for humanoid robots, virtual motion for digital actors and mannequins, and natural motions of human beings.
He teaches Robotics at Ecole Normale Supérieure in Paris. He has edited three books. He has published more than 150 papers in international journals and conferences in Robotics, Computer Science, Automatic Control and recently in Neurosciences. He has been the 2011-2012 recipient of the Chaire Innovation technologique Liliane Bettencourt at Collège de France in Paris. His current project Actanthrope (ERC-ADG 340050) is devoted to the computational foundations of anthropomorphic action.
|
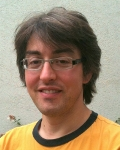 |
Nicolas Mansard
Senior Researcher, CNRS-LAAS, Toulouse, France.
|
What Is Optimal in Robot Motion?
Optimal control provides an appealing theoretical framework to design, generate and control complex movements on any robotic system. However, the automatic generation of a trajectory from an optimal-control problem is often a costly mathematical process. As roboticists we then rely on two approximations to fasten the resolution. First we accept sub-optimality; then we design dedicated heuristics: simplified models (e.g. table cart in locomotion), warm-start assumptions (e.g. motion graphs in computer animation), problem reformulation based on non-trivial properties (e.g. flatness in quadcopters). In this presentation, we will discuss the trade-off between these choices, and how we can imagine to go beyond them. The presentation will be based on the context of locomotion in complex nonflat terrains.
|
Nicolas Mansard is a permanent researcher at LAAS-CNRS, Toulouse since October 2008. He is involved in the Gepetto research group, with Philippe Souères, Florent Lamiraux, Olivier Stasse and Jean-Paul Laumond.
His research activities are concerned with sensor-based control, and more specifically the integration of sensor-based schemes into humanoid robot applications. It is an exciting research topic at the intersection of the fields of robotics, automatic control, signal processing and numerical mathematics. His main application field is currently the Humanoid robotics, as it causes serious challenges that are representative of many other robot domains.
Before being in the Gepetto group, He was a post-doc in Tsukuba, and a post-doc at the IA Lab of Stanford University, in the group led by Prof.O.Khatib. Nicolas Mansard has done his PhD. with Francois Chaumette in the Lagadic group, at INRIA Rennes. He was a student of the École Normale Supérieure de Bretagne and did both MSc (Robotics) and BEng (CS) in the INP Grenoble (ENSIMAG). |
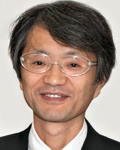 |
Yoshihiko Nakamura
Professor, University of Tokyo, Japan
|
NOH: The Dance that Hides Movements
Noh originates form Gigaku, drama-dance performance, that arrived Japan from China in the 7th century. Kan'ami and his son Zeami in the 14th century established the style of Noh in the way we see today. The dance performance is highly abstracted and express itself by the minimum movements. It is also characterized by a mask that the performer wears and expresses by its slight inclination. It is also known that a performance always accompanies with a Noh text. We have had an opportunity to motion-capture a young professional performer, Keisuke Shiozu, in Kita-School with his fellow musicians. We have analyzed the performer's movements by the musculoskeletal analysis. We are also investigating the dialog between the musicians and the dancer in the performance. This presentation will show the preliminary results of the analysis.
|
Yoshihiko Nakamura is Professor at Department of Mechano-Informatics, University of Tokyo. He received Doctor of Engineering Degree from Kyoto University. Humanoid robotics, cognitive robotics, neuro musculoskeletal human modeling, biomedical systems, and their computational algorithms are his current fields of research. He is Fellow of JSME, Fellow of RSJ, Fellow of IEEE, and Fellow of WAAS. Dr. Nakamura serves as President of IFToMM (2012-2015). Dr. Nakamura is Foreign Member of Academy of Engineering Science of Serbia, and TUM Distinguished Affiliated Professor of Technische Universität München.
|
 |
Edouard Pauwels
Associated Professor, IRIT, University of Toulouse
|
Linear conic optimization for inverse optimal control
The presentation is dedicated to the problem of Lagrangian identification based on observed trajectories. We follow the principle of inverse optimality in the context of free terminal time optimal control. The problem will be formulated based on the notions of occupation measures, complementarity in linear programming and relaxation of Hamilton-Jacobi-Bellman equation. Important properties of this approach from the optimal, numerical and statistical point of view will be described. In particular, these ideas are amenable to numerical approximations and we will present simulations on accademic examples and preliminary results in the context of human locomotion.
|
Edouard Pauwels is Associated Professor at University of Toulouse. His research interests evolve around numerical optimization with applications to machine learning, robotics and computational biology. He got his PhD November 2013 at Center for computational biology in Mines ParisTech working on the application of machine learning techniques in biological contexts. During a Postdoc in MAC team at LAAS-CNRS, Toulouse, he studied the application of polynomial optimization to direct and inverse optimal control. From 2014 to 2015 he was a Postdoc in the Operation Research Group in the Faculty of Industrial Engineering and Management in Technion, Israel, working on large scale convex optimization.
|
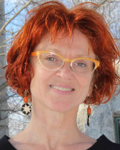 |
Dagmar Sternad
Professor, Northeastern University, Departments of Biology, Electrical & Computer Engineering, and Physics, Boston, USA
|
Control of intermittent and continuous interactions with objects.
How do humans manage their actions and interactions with the physical world? How do we learn new skills or re-learn basic behaviors after injury, such as reaching to drink from a glass without spilling? Our research approach analyzes how task dynamics constrain and enable interactions and their improvement with practice. Starting with a mechanical model of the task, humans interactively execute this task in a virtual environment. Based on mathematical analyses of the model, we study how humans develop stable solutions to meet complex task demands including manipulating underactuated objects. Key concepts in our analysis are variability, stability, and predictability. Using three model tasks, throwing a ball, rhythmic bouncing of a ball, and transporting a “cup of coffee”, we show that humans develop skill by: 1) exploiting solutions with dynamic stability, 2) finding error-tolerant strategies and channeling noise into task-irrelevant dimensions, 3) optimizing predictability of object dynamics. These findings set the stage for developing propositions about the controller in terms of dynamic primitives.
|
Dagmar Sternad received her BS in Movement Science and Linguistics from the Technical University of Munich and her PhD in Experimental Psychology from the University of Connecticut. From 1995 until 2008, she was Assistant, Associate, and Full Professor at the Pennsylvania State University in Kinesiology and Integrative Biosciences. Since 2008, she holds an interdisciplinary appointment in the departments of Biology, Electrical and Computer Engineering, and Physics at Northeastern University in Boston. Her research in computational neuroscience and motor control focuses on learning and control of sensorimotor coordination in humans, both in healthy and neurologically impaired individuals. This work spans behavioral experiments with mathematical models of control and nonlinear dynamics, bridging biology with engineering and physics. The results are documented in over 100 publications in scientific journals and several books. She holds editorial appointments in several journals, including 9 years of executive editor of the Journal of Motor Behavior, and has been member of NIH and NSF grant review panels. Her research has been continuously supported by the National Institute of Health, National Science Foundation, and American Heart Association and others.
|
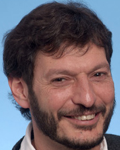 |
Mario Sznaier
Professor, Electrical and Computer Engineering Department, Northeastern University, Boston, USA
|
The interplay between Big Data and sparsity in system theory
Arguably, one of the hardest challenges faced now by the systems community stems from the exponential explosion in the availability of data, fueled by recent advances in sensing and actuation capabilities. Simply stated, classical techniques are ill equipped to handle very large volumes of (heterogeneous) data, due to poor scaling properties and to impose the structural constraints required to implement ubiquitous sensing and control. The goal of this talk is to explore how this “curse of dimensionality” can be potentially overcome by exploiting the twin “blessings” of self-similarity (high degree of spatio-temporal correlation in the data) and concentration of measure (inherent underlying sparsity). While these ideas have already been recently used in machine learning (for instance in the context of dimensionality reduction and variable selection), they have hitherto not been fully exploited in systems theory. By appealing to a deep connection to semi-algebraic geometry, rank minimization and matrix completion we will argue that, in the context of systems theory, the limiting factor is given by the ``memory" of the system (as defined by the tree-width of an underlying graph) rather than the size of the data itself, and discuss the implications of this fact. These concepts will be illustrated examining examples of "easy" and "hard" problems, including the synthesis of filters and controllers subject to information flow constraints, and identification of classes of non-linear systems. The talk will conclude with an application of these ideas to the non-trivial problem of extracting actionable information from very large data streams. In particular, we will show how exploiting sparsity leads to tractable, scalable solutions to the problems of anomaly detection and activity analysis from video streams.
|
Mario Sznaier is currently the Dennis Picard Chaired Professor at the Electrical and Computer Engineering Department, Northeastern University, Boston. Prior to joining Northeastern University, Dr. Sznaier was a Professor of Electrical Engineering at the Pennsylvania State University and also held visiting positions at the California Institute of Technology. His research interest include robust identification and control of hybrid systems, robust optimization, and dynamical vision. Dr. Sznaier is currently serving as an associate editor for the journal Automatica, chair of the IEEE Control Systems Society Technical Committee on Computational Aspects of Control Systems Design, General Chair of the 2016 IEEE Multi Systems Conference and Program Chair of the 2017 IEEE Conference on Decision and Control. Additional recent service includes Executive Director of the IEEE CSS (2007-2011), member of the Board of Governors of the CSS (2006-2014), General Program Chair of the 2009 IFAC Symposium on Robust Control Design, and Program vice-chair of the 2008 IEEE Conf. on Decision and Control. Dr. Sznaier was a plenary speaker at the 2012 IFAC Symposium on Robust Control Design, 2012 IFAC Symposium on System Identification, the 2012 Mediterranean Control Conference and the 2013 RPIC, and delivered a Semi-Plenary lecture at the 2012 IEEE Conference on Decision and Control. In 2012 he received a distinguished member award from the IEEE Control Systems Society for his contributions to robust control, identification and dynamic vision. A list of publications and current research projects can be found at http://robustsystems.coe.neu.edu
|
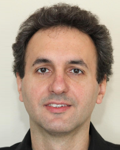 |
Emanuel Todorov
Associate Professor, University of Washington, Movement Control Laboratory, Seattle, USA
|
Synthesis of contact-rich behaviors with optimal control
Animals and machines interact with their environment mainly through physical contact. Yet the discontinuous nature of contact dynamics complicates planning and control, especially when combined with uncertainty. We have recently made progress in terms of optimizing complex trajectories that involve many contact events. These events do not need to be specified in advance, but instead are discovered fully automatically. Key to our success is the development of new models of contact dynamics, which enable continuation methods that in turn help the optimizer avoid a combinatorial search over contact configurations. We can presently synthesize humanoid trajectories in tasks such as getting up from the floor, walking and running, turning, riding a unicycle, as well as a variety of dexterous hand manipulation tasks. When augmented with warm-starts in the context of model predictive control, our optimizers can run in real-time and be used as approximately-optimal feedback controllers. Some of these controllers have already been transferred to physical robots, via ensemble optimization methods that increase robustness to modeling errors. The resulting trajectory libraries are also used to guide the training of recurrent neural networks. After training (in the cloud) the networks can control the body autonomously, without further help from the trajectory optimizer.
|
Emmanuel Todorov, Associate Professor, graduated from MIT in 1998 with a PhD in Cognitive Neuroscience. He joined the University of Washington from the Department of Cognitive Science at the University of California San Diego.
Todorov’s research focus is intelligent control in biological and artificial systems. He combines experimental and computational methods to investigate how the brain controls the body in a variety of motor tasks. He also develops biologically-inspired control algorithms which aim to solve complex problems beyond the reach of traditional control theory.
|
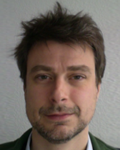 |
Pierre-Brice Wieber
Chargé de Recherche, INRIA, Grenoble, France.
|
Geometric and numerical aspects of redundancy
When a robot has more than one way to realize a given objective, the general approach is to leverage this redundancy and introduce additional objectives, that the robot could try to satisfy at the same time. A point which is often overlooked here, is that the degree of redundancy of a robot with respect to a given set of objectives can vary, depending on the configuration of the robot and of its objectives. This is an important question since it drives how much the different objectives can be realized by the robot, depending on the situation. Measuring this degree of redundancy is usually done with the rank of the Jacobian matrix between the configuration space of the robot and the space of objectives. But this approach is not reliable in general, and to understand why leads us to investigate when a linear approximation of a nonlinear model is meaningful or not, conjuring various geometric and numerical aspects of redundancy.
|
Pierre-Brice Wieber has been Chargé de Recherche at INRIA Grenoble Rhône-Alpes in the Bipop team since 2001. His primary research interest is in optimization methods for humanoid and industrial robot control. He obtained a Ph.D. degree in Robotics from École des Mines de Paris in 2000, and has been a visiting researcher at CNRS/AIST JRL in Tsukuba, Japan, as a JSPS fellow in 2005, and as a Marie Curie International Outgoing fellow in 2008-2010. He is Associate Editor for IEEE Transactions on Robotics since 2014, and currently participates in the French project Romeo 2 and the European project Comanoid. |
|